Entertainment
Machine Learning: Revolutionizing Technology and Innovation
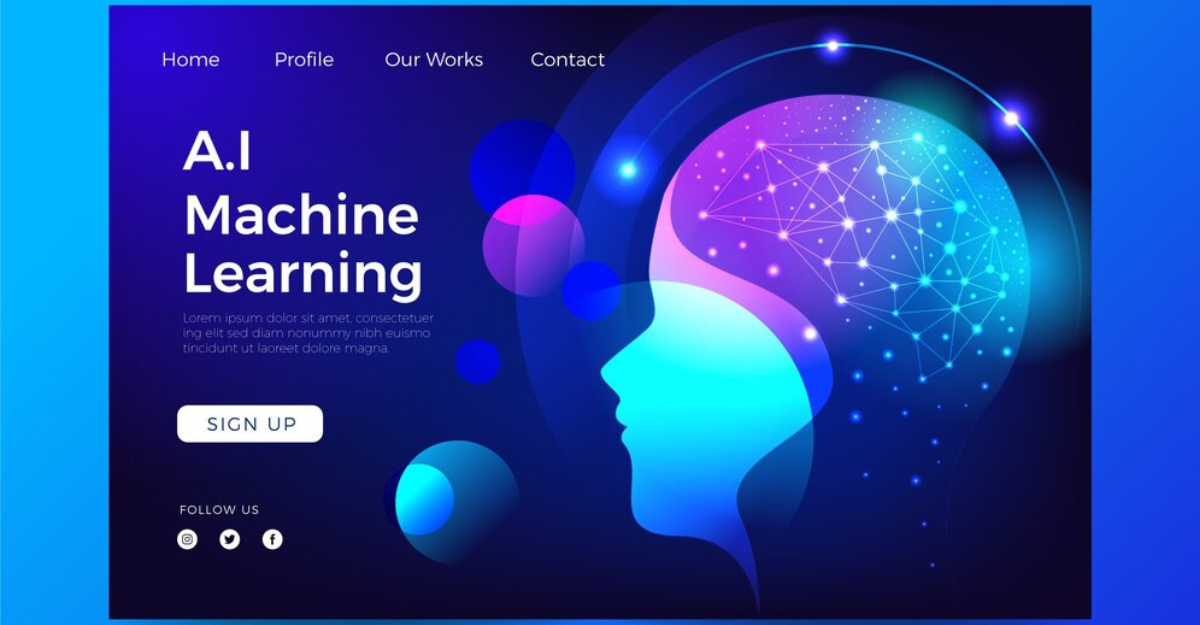
Machine Learning (ML) is one of the most transformative technologies of the 21st century. It is a subset of artificial intelligence (AI) that enables systems to learn from data and make decisions or predictions without explicit programming. From personalized recommendations to autonomous vehicles, ML is reshaping industries and improving how we interact with technology.
How Machine Learning Works
The foundation of ML lies in algorithms and data. Here’s a step-by-step overview of how it operates:
- Data Collection: ML systems require vast amounts of data, which could be historical records, images, or real-time inputs.
- Data Processing: Collected data is cleaned and structured to remove inconsistencies and ensure its usability.
- Algorithm Training: A model is trained using selected ML algorithms to recognize patterns and correlations in the data.
- Testing and Validation: The model is tested on unseen data to evaluate its performance and accuracy.
- Deployment: Once optimized, the ML model is deployed in real-world applications to generate predictions or automate tasks.
This cyclical process ensures that ML systems improve over time as they encounter more data.
Types of Machine Learning
ML can be categorized into three main types based on how algorithms learn from data:
Supervised Learning
In supervised learning, the model is trained on labeled data where inputs and corresponding outputs are known. For example, predicting house prices based on features like location and size relies on supervised learning.
2. Unsupervised Learning
Unsupervised learning deals with unlabeled data. The system identifies patterns and clusters within the data without predefined outputs. It’s often used for market segmentation and anomaly detection.
3. Reinforcement Learning
This approach involves learning through trial and error. The system receives rewards for correct actions and penalties for mistakes, optimizing its behavior over time. Reinforcement learning powers innovations like robotic control and game-playing AI.
Applications of Machine Learning
The versatility of ML has led to its adoption across numerous industries. Below are some of its most impactful applications:
Healthcare
ML is revolutionizing healthcare by enabling early disease detection, personalized treatment, and efficient patient care. For example, ML models analyze medical images to identify abnormalities like tumors with high accuracy.
Finance
In the finance sector, ML algorithms detect fraudulent transactions, assess credit risks, and automate trading decisions. These capabilities improve efficiency and enhance security.
Retail and E-commerce
ML enhances the shopping experience through personalized recommendations, dynamic pricing, and inventory management. Platforms like Amazon and Netflix leverage ML to suggest products or content tailored to individual preferences.
Transportation
From optimizing delivery routes to powering self-driving cars, ML is transforming the transportation industry. Autonomous vehicles, in particular, rely heavily on ML to interpret surroundings and make real-time decisions.
Education
ML-driven tools are personalizing education by adapting content to suit each learner’s needs. Additionally, automated grading systems and virtual tutors improve accessibility and reduce workload for educators.
Benefits of Machine Learning
ML offers several advantages that make it indispensable for businesses and individuals alike:
Automation of Tasks
ML eliminates repetitive tasks by automating processes, allowing professionals to focus on higher-value activities.
Improved Accuracy
By analyzing massive datasets, ML models can achieve remarkable accuracy in tasks like diagnosing diseases or predicting trends.
Scalability
ML systems can handle vast amounts of data and adapt to growing demands, making them suitable for industries with rapidly expanding datasets.
Challenges of Machine Learning
Despite its immense potential, ML faces several challenges that need to be addressed:
Data Quality Issues
ML models are only as good as the data they are trained on. Inaccurate or biased data can lead to flawed predictions.
Computational Requirements
Training ML models requires significant computational resources, which can be costly and environmentally taxing.
Ethical Concerns
ML raises questions about data privacy, security, and potential misuse. Ensuring ethical practices is crucial for its responsible deployment.
Future of Machine Learning
The future of ML is promising, with advancements continuing to push its boundaries. Emerging trends include:
- Explainable AI: Enhancing transparency by allowing users to understand how ML models make decisions.
- Edge Computing: Bringing ML capabilities closer to users by processing data locally, reducing latency.
- Quantum Machine Learning: Leveraging quantum computing to solve complex ML problems more efficiently.
These developments signal a future where ML becomes even more integral to our lives.
Conclusion
More than just a technical advance, machine learning is a catalyst for innovation in almost every sector of the economy. Machine learning (ML) is making systems smarter and changing how we live and work by learning from data and adjusting to new problems. The potential of machine learning to solve global issues and open up new avenues is boundless as we continue to investigate its potential.
FAQs
Q: What is Machine Learning used for?
Machine Learning is used for tasks such as image recognition, fraud detection, personalized recommendations, and automating complex processes.
Q: How is Machine Learning different from Artificial Intelligence?
Machine Learning is a subset of AI focused on creating systems that learn from data, while AI encompasses a broader range of intelligent behaviors, including reasoning and problem-solving.
Q: What skills are needed to work in Machine Learning?
Key skills include programming (e.g., Python, R), knowledge of algorithms, statistical analysis, and familiarity with tools like TensorFlow or PyTorch.
Q: Can Machine Learning models replace humans?
While ML can automate many tasks, it is designed to augment human capabilities, not replace them entirely.
Q: What industries benefit most from Machine Learning?
Industries like healthcare, finance, retail, transportation, and education are among those benefiting significantly from ML innovations.
Entertainment
Incestflix: Understanding the Controversy and Its Implications
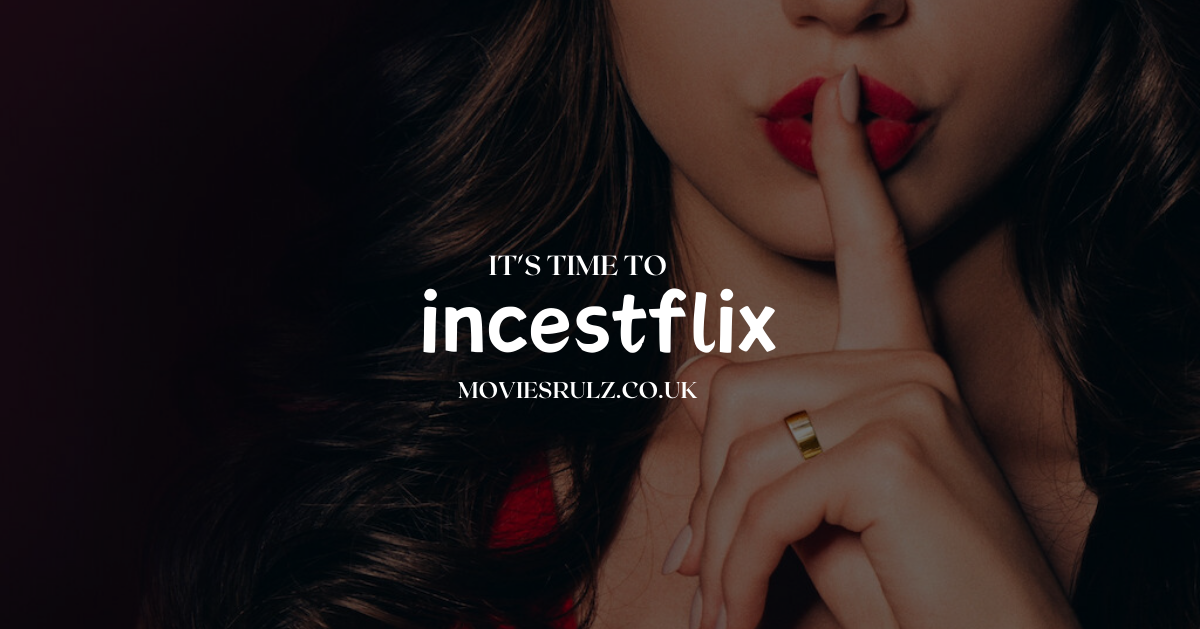
Incestflix is a term that has sparked widespread controversy, discussions, and debates across various platforms. Though it’s often associated with taboo or sensitive content, the deeper conversation surrounding it involves ethical, psychological, and legal dimensions that society continues to grapple with.
The Origins of Incestflix
The term “Incestflix” gained traction as a nickname for certain media outlets or platforms that allegedly promote or display taboo content. It’s a blend of “incest” and “Netflix,” which has inadvertently contributed to its notoriety.
Why Is Incestflix Controversial?
The controversy stems from the association with content considered socially and morally unacceptable. Many argue it trivializes serious issues and raises concerns about normalization through media exposure.
The Role of Media in Addressing Sensitive Topics
Media has an undeniable influence on shaping public opinions. Platforms like Incestflix, whether real or perceived, raise questions about how much responsibility media holds when addressing or showcasing sensitive content.
Ethical Implications of Taboo Content
Ethics play a crucial role in this discussion. The portrayal of taboo subjects like incest in media leads to polarizing views. Supporters argue for creative freedom, while opponents raise alarms over moral degradation.
Psychological Effects on Viewers
Repeated exposure to sensitive content can desensitize individuals, potentially blurring the lines between reality and fiction. This raises concerns about the mental health implications for impressionable audiences.
Legal Concerns and Censorship
Many countries have strict laws against the depiction of incestuous relationships, even in fictional media. Platforms hosting such content face risks of being banned or heavily regulated.
Cultural Differences in Perception
Cultural norms significantly influence the acceptance or rejection of taboo subjects in media. What’s considered unacceptable in one society might not face the same level of scrutiny in another.
The Thin Line Between Art and Exploitation
Creators often defend their work as art, arguing it’s meant to provoke thought or challenge norms. However, critics view such content as exploitative and harmful.
How Public Opinion Shapes Platform Policies
Public backlash often pushes platforms to revise their policies. Incestflix has sparked numerous debates, leading some platforms to impose stricter guidelines on the content they host.
The Importance of Parental Controls
Given the accessibility of digital platforms, parental controls are essential. They provide a way for guardians to shield younger audiences from inappropriate content.
Alternatives to Consuming Controversial Content
For those seeking thought-provoking media without crossing moral boundaries, numerous platforms offer ethically grounded stories that are equally engaging.
Educating Viewers About Responsible Consumption
Education is key to understanding the potential impact of what we consume. Platforms should invest in awareness campaigns to help users make informed choices.
Legal Actions Against Content Violations
Authorities worldwide are increasingly vigilant about monitoring and taking down content that violates societal norms and legal boundaries.
How Incestflix Reflects Broader Media Trends
The rise of controversial platforms like Incestflix reflects a broader trend in media, where shock value often takes precedence over meaningful storytelling.
Conclusion
The debate surrounding Incestflix is more than just about a term or platform; it’s a reflection of societal values, cultural norms, and the evolving dynamics of media consumption. While creative freedom is essential, it must be balanced with ethical considerations and responsibility toward viewers.
FAQs
What is the main concern with Incestflix?
The main concern revolves around the ethical and moral implications of the content it represents and its potential influence on societal values.
Why do some people defend taboo content in media?
Defenders often cite artistic freedom and the need to address uncomfortable topics to spark meaningful dialogue.
Are there legal repercussions for hosting such content?
Yes, many countries impose strict legal actions against platforms that violate societal norms or legal standards.
How can viewers protect themselves from inappropriate content?
Using parental controls, content filters, and being mindful of viewing habits can help protect against inappropriate media exposure.
What lessons can media platforms learn from this controversy?
Platforms should prioritize ethical responsibility, transparency, and audience awareness while balancing creative freedom
Entertainment
Vhsgjqm: Exploring the Significance and Impact
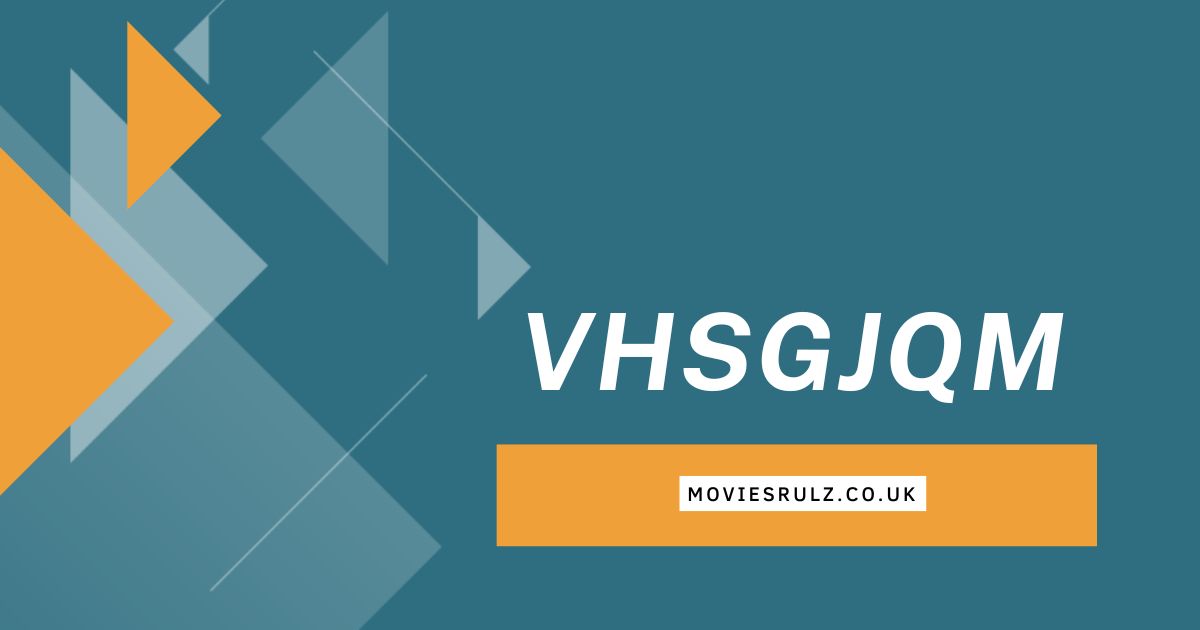
Vhsgjqm is a unique and intriguing topic that sparks curiosity. While its roots might appear enigmatic, understanding this concept involves delving into its background and implications in various contexts.
The Origin and Meaning of Vhsgjqm
The term vhsgjqm may have niche origins or could be related to a specific field. Exploring its etymology sheds light on its essence and purpose.
Applications of Vhsgjqm in Modern Contexts
The versatility of vhsgjqm allows it to integrate seamlessly into diverse domains. Its adaptability is one of the factors contributing to its growing prominence.
Why Vhsgjqm Is Gaining Popularity
In a world constantly evolving, vhsgjqm offers innovative solutions and perspectives. It resonates with audiences who seek forward-thinking ideas and practical implementations.Businesses leveraging vhsgjqm strategies often outpace competitors
How Vhsgjqm Influences Daily Lives
Though it might seem distant from everyday scenarios, vhsgjqm significantly shapes how we interact with the world. From technology to personal development, its influence is undeniable.
Technological Advancements Related to Vhsgjqm
Technological strides have intertwined with vhsgjqm, making it a cornerstone in fields like AI, automation, and sustainable practices.
The Role of Vhsgjqm in Education
Educational frameworks are increasingly adopting vhsgjqm principles to foster innovative thinking and problem-solving skills in students.
Business Implications of Vhsgjqm
Businesses leveraging vhsgjqm strategies often outpace competitors. It offers a roadmap to efficiency, growth, and sustainability.
Environmental Connections of Vhsgjqm
In environmental science, vhsgjqm contributes to developing sustainable practices and solutions for pressing global issues.
Cultural Impact of Vhsgjqm
Cultural landscapes are adapting vhsgjqm in artistic and social expressions, fostering inclusivity and creativity.
Challenges Associated with Vhsgjqm
Despite its benefits, vhsgjqm faces challenges such as resistance to change and implementation hurdles.
The Future of Vhsgjqm
The potential of vhsgjqm is vast, with advancements anticipated in multiple sectors, promising a transformative future.
Personal Development and Vhsgjqm
On an individual level, vhsgjqm can enhance personal growth by encouraging adaptability and forward-thinking.
Steps to Embrace Vhsgjqm in Your Life
Implementing vhsgjqm principles can be a straightforward process with clear steps to integrate its benefits.
The Social Implications of Vhsgjqm
Vhsgjqm has also found a place in shaping social dynamics. It fosters collaboration and inclusivity by encouraging diverse perspectives and shared problem-solving approaches. This concept often bridges gaps between traditional and modern methodologies, uniting communities toward common goals. Whether in team environments or broader societal initiatives, vhsgjqm offers frameworks that promote harmony and innovation, making it a critical tool in navigating today’s interconnected world.
Vhsgjqm’s Role in Shaping Global Trends
On a global scale, vhsgjqm is driving trends that emphasize sustainability, efficiency, and forward-thinking solutions. Countries and organizations are adopting its principles to address challenges like climate change, economic disparities, and technological integration. By serving as a unifying force across industries and borders, vhsgjqm not only addresses present-day challenges but also lays the foundation for a more equitable and resilient future. Its impact is undeniable, transforming the way the world approaches progress and growth.
Conclusion
Vhsgjqm is more than just a concept; it’s a movement that influences various facets of life. By embracing it, individuals and organizations alike can unlock new opportunities for success and growth.
FAQs
What does vhsgjqm stand for?
The specific meaning of vhsgjqm depends on its application but generally represents innovation and adaptability.
How can vhsgjqm benefit businesses?
Vhsgjqm enhances efficiency, promotes sustainable practices, and helps businesses remain competitive.
Is vhsgjqm relevant in personal development?
Absolutely. It encourages adaptability, innovative thinking, and problem-solving.
What are the challenges of adopting vhsgjqm?
Common challenges include resistance to change and the need for strategic implementation.
What is the future of vhsgjqm?
Vhsgjqm has a promising future with advancements expected in technology, education, and sustainability.
Entertainment
Bossb1tch0680: Empowering the Digital Age with Confidence and Style
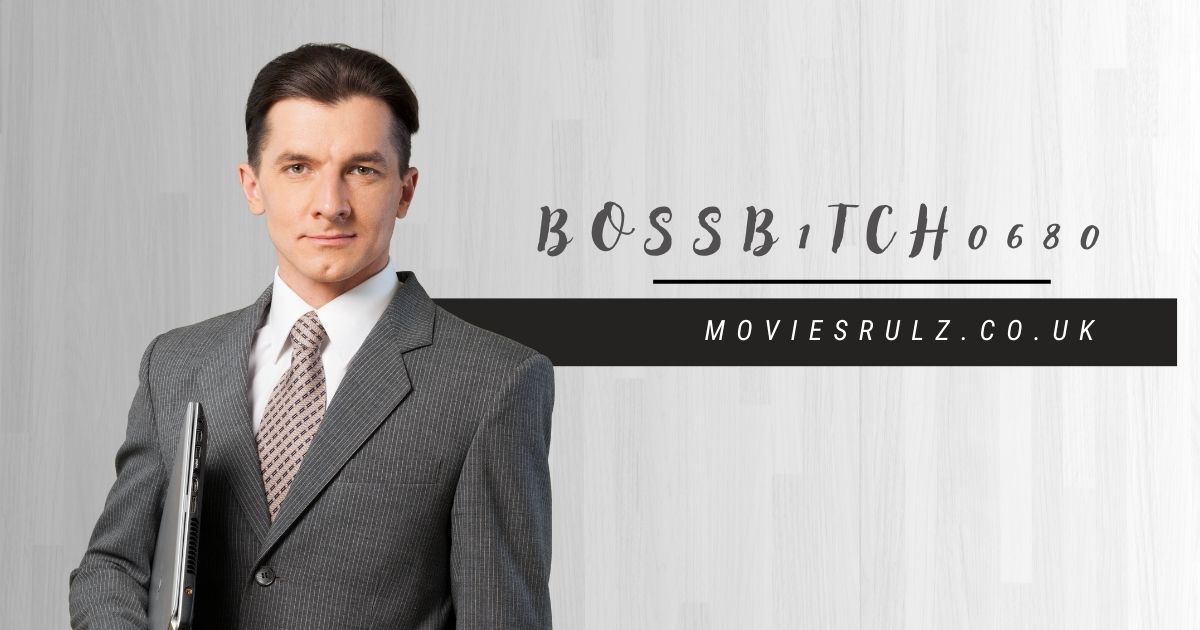
In a world where digital presence is synonymous with personal identity, individuals are carving out unique spaces for themselves. Among them, “Bossb1tch0680” stands out as a beacon of confidence, resilience, and creativity. This persona encapsulates the energy of taking charge, breaking barriers, and owning one’s narrative.
What Does Bossb1tch0680 Represent?
The name itself exudes power and individuality. “Bossb1tch0680” isn’t just a username; it’s a statement. It represents a person who is unapologetically themselves, fiercely independent, and determined to create their own path.
The Power of Online Identity
In today’s interconnected world, an online identity is as vital as a physical one. Platforms like Instagram, TikTok, and Twitter allow individuals to express themselves, and Bossb1tch0680 symbolizes the freedom to craft a powerful and unique identity in the digital age.
Confidence in the Digital World
Confidence is key to thriving online. With millions of voices clamoring for attention, Bossb1tch0680 teaches us that standing tall and embracing our quirks is the ultimate strength. Whether through bold posts or fearless opinions, confidence is the cornerstone of influence.
Building a Personal Brand
Personal branding is no longer exclusive to businesses or celebrities. It’s about showcasing what makes you unique. Bossb1tch0680 shows us how a strong, consistent brand can resonate with followers and inspire them.
Social Media as a Tool for Influence
Social media isn’t just a place to post selfies; it’s a powerful tool to reach audiences worldwide. By strategically using these platforms, Bossb1tch0680 proves how one can amplify their voice and make a difference.
Breaking Gender Stereotypes
In a society still grappling with gender norms, Bossb1tch0680 defies expectations. The persona challenges stereotypes, proving that being bold, assertive, and ambitious isn’t limited by gender.
Inspiring Others Through Content
Content is king—or queen. From motivational posts to creative projects, Bossb1tch0680 inspires a generation to be true to themselves. The ability to impact lives through relatable content is a testament to the power of digital storytelling.
Challenges in Being Bold Online
Being bold comes with its fair share of challenges. Critics, trolls, and haters are part of the package. However, the courage to stay authentic amidst adversity is what sets Bossb1tch068’0 apart.
Overcoming Criticism and Negativity
Online spaces can often feel hostile, but resilience is key. Bossb1tch068’0 demonstrates that overcoming negativity requires focusing on one’s mission and ignoring unnecessary noise.
Balancing Authenticity and Professionalism
While authenticity attracts followers, professionalism builds trust. Bossb1tch068’0 strikes the perfect balance by being genuine while maintaining a polished and respectful approach.
The Importance of a Supportive Community
No one succeeds alone. A supportive community of like-minded individuals is crucial. Bossb1tch0680 highlights the significance of surrounding oneself with people who uplift and encourage.
Lessons From Bossb1tch0680’s Success
What can we learn from this bold persona? Persistence, adaptability, and staying true to oneself are the keys to building a memorable and impactful digital presence.
Adopting a Growth Mindset
The journey of personal growth never ends. Bossb1tch068’0 inspires us to embrace challenges as opportunities and continuously evolve.
Conclusion
Bossb1tch068’0 isn’t just a username; it’s a movement. It’s about embracing confidence, authenticity, and resilience. In a world full of followers, be a boss—take charge of your story and inspire others along the way.
FAQs
What does the name Bossb1tch0680 mean?
It symbolizes power, independence, and unapologetic authenticity.
How can I create a unique online persona?
Start by being authentic, consistent, and focusing on your strengths.
What are the challenges of being bold online?
Facing criticism and negativity is common, but resilience helps overcome these challenges.
How important is community in online success?
A supportive community is crucial for growth and motivation.
What’s the main lesson from Bossb1tch0680?
Stay true to yourself, embrace confidence, and never stop growing.
-
Sports3 weeks ago
Viprow.us.com Odds: Understanding Sports Betting Benefits
-
Entertainment3 weeks ago
MoviesRulz: Your Ultimate Guide to Streaming Blockbusters Online
-
Technology1 month ago
Drone Review Miracoup: Unveiling Its Features and Performance
-
Entertainment1 month ago
Nhentai.nef: Understanding Its Role and Impact in Digital Media
-
Buisness1 month ago
LessInvest: How to Maximize Returns with Minimal Investment
-
Entertainment1 month ago
Discover Moviee07.vip: A Complete Guide to Free Online Streaming
-
General1 month ago
Success100x.com Goals: Achieve Success with Strategic Vision
-
Life style1 month ago
Vivianloantran: Discovering a Rising Name with Impact